High-quality labeled data is the foundation of AI models’ performance. Data annotation companies play a crucial role in delivering this data, but as demand grows, traditional manual processes struggle to keep up. With technology, companies can scale quickly and streamline their operations.
Let’s see how technology can help teams with the data annotation process, streamline operations, and help manage common challenges.
Why Technology is Critical for Data Annotation
The need for annotated data is growing exponentially as AI applications become more advanced. While human annotators are essential for complex tasks, relying solely on manual efforts creates significant challenges:
- Time-Consuming Processes. Manual annotation can’t keep up with large-scale datasets.
- Human Errors. Fatigue and repetitive tasks lead to inconsistencies.
- Scalability Issues. Increasing the workforce to handle demand raises costs.
For any data annotation company, leveraging technology is now a necessity. Tools like AI-assisted platforms and automation systems bridge these gaps by speeding up annotation, improving accuracy, and enabling companies to scale without sacrificing quality.
Key Technologies Transforming Data Annotation
Modern data annotation companies use several technologies to enhance productivity and ensure precision.
AI-Assisted Annotation Tools
AI-assisted tools pre-label datasets to reduce manual effort. For example:
- In image annotation, AI identifies objects like cars or trees and creates bounding boxes.
- In-text annotation, AI highlights named entities by highlighting names, dates, and locations.
These tools can auto-annotate simple data points quickly, allowing human annotators to focus on refining complex or ambiguous inputs. This collaboration ensures high accuracy while speeding up the overall workflow.
Benefits:
- Speeds up annotation workflows.
- Reduces repetitive tasks for human annotators.
- Minimizes costs for large-scale projects.
Automation Platforms
Automation platforms streamline repetitive annotation tasks and provide project management capabilities.
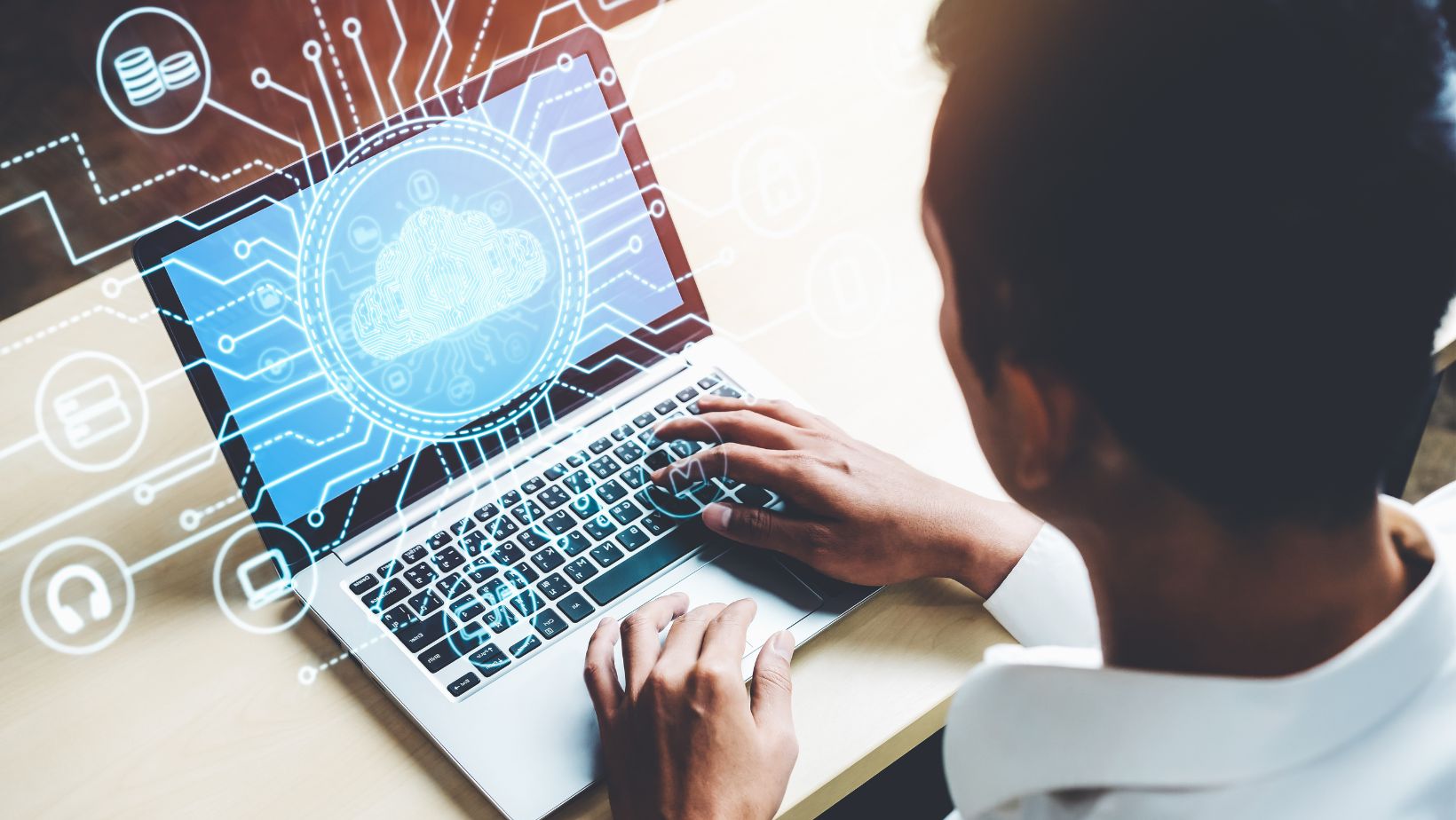
Key features include:
- Bulk Annotation. Annotate large datasets quickly by applying consistent rules.
- Task Allocation. Automatically assign tasks to annotators based on skills or workload.
- Error Detection. Platforms flag inconsistent labels for review.
These platforms allow companies to scale efficiently, assisting with saving time and providing accurate output.
Quality Control Technologies
Maintaining annotation quality is essential for effective AI training. Machine learning-based tools help monitor quality through:
- Error Detection. Identifies mislabeled or ambiguous data points.
- Gold-Standard Benchmarks. Compares annotations against pre-approved datasets.
- Outlier Detection. Flags data that doesn’t align with expected patterns.
Automating quality checks ensures that data annotations remain accurate, consistent, and reliable for AI model training. These tools reduce the need for manual reviews and designing frameworks for the standard.
Cloud-Based Annotation Platforms
Cloud platforms have transformed collaboration in data annotation, especially for remote teams.
Advantages:
- Real-Time Collaboration. Multiple annotators can work simultaneously on the same dataset.
- Secure Access. Platforms manage role-based access to protect sensitive data.
- Scalability. Cloud systems adapt to handle growing datasets without performance issues.
Cloud-based annotation platforms make it easier to manage distributed teams, track project progress, and maintain security.
Balancing AI and Human Input for Accuracy
While automation tools have revolutionized data annotation, human expertise remains irreplaceable. Technology and humans work together to deliver the best results.
The role of annotating AI systems is to automate repetitive, low-complexity tasks such as labeling basic objects or identifying patterns. Human annotators step in to review, refine, and handle more nuanced edge cases.
Task | AI Role | Human Role |
Simple, Repetitive Tasks | Automates labeling (e.g., bounding boxes) | Reviews and corrects AI outputs. |
Complex Data | Pre-labels where possible | Handles edge cases and ambiguities. |
Quality Control | Detects errors and anomalies | Verifies flagged issues manually. |
Using data annotation AI, companies can refine their activities, automate repetitive patterns, and leverage strategic activities. Such human-in-the-loop (HITL) approach is faster and more accurate, considering the needs of AI model development.
Example: In self-driving car datasets, AI can pre-label vehicles and road signs. Humans then validate edge cases, such as pedestrians in unusual positions or obstructed objects.
The Impact of Technology on Scalability and Cost
Technology enables data annotation companies to scale operations while keeping costs manageable.
How Technology Reduces Costs and Time
- Automated Annotation. It helps to reduce the time that specialists spend on repetitive tasks.
- Optimized Workflows. Automation platforms save time through task allocation and error detection.
- Cloud Scalability. Handles larger datasets without additional infrastructure costs.
AI tools play a key role in tasks like object detection, where large-scale datasets require annotating objects such as vehicles, pedestrians, or products. Automation helps pre-label these objects efficiently, and human annotators refine the results to ensure accuracy.
Scenario: Annotating thousands of medical images manually would take months and significant resources. AI tools can pre-label tumors or abnormalities, and human experts verify the results, significantly reducing costs and time.
Ensuring Data Security and Privacy with Technology
Data annotation quite often deals with personal and sensitive information, which requires additional private and secure measures.
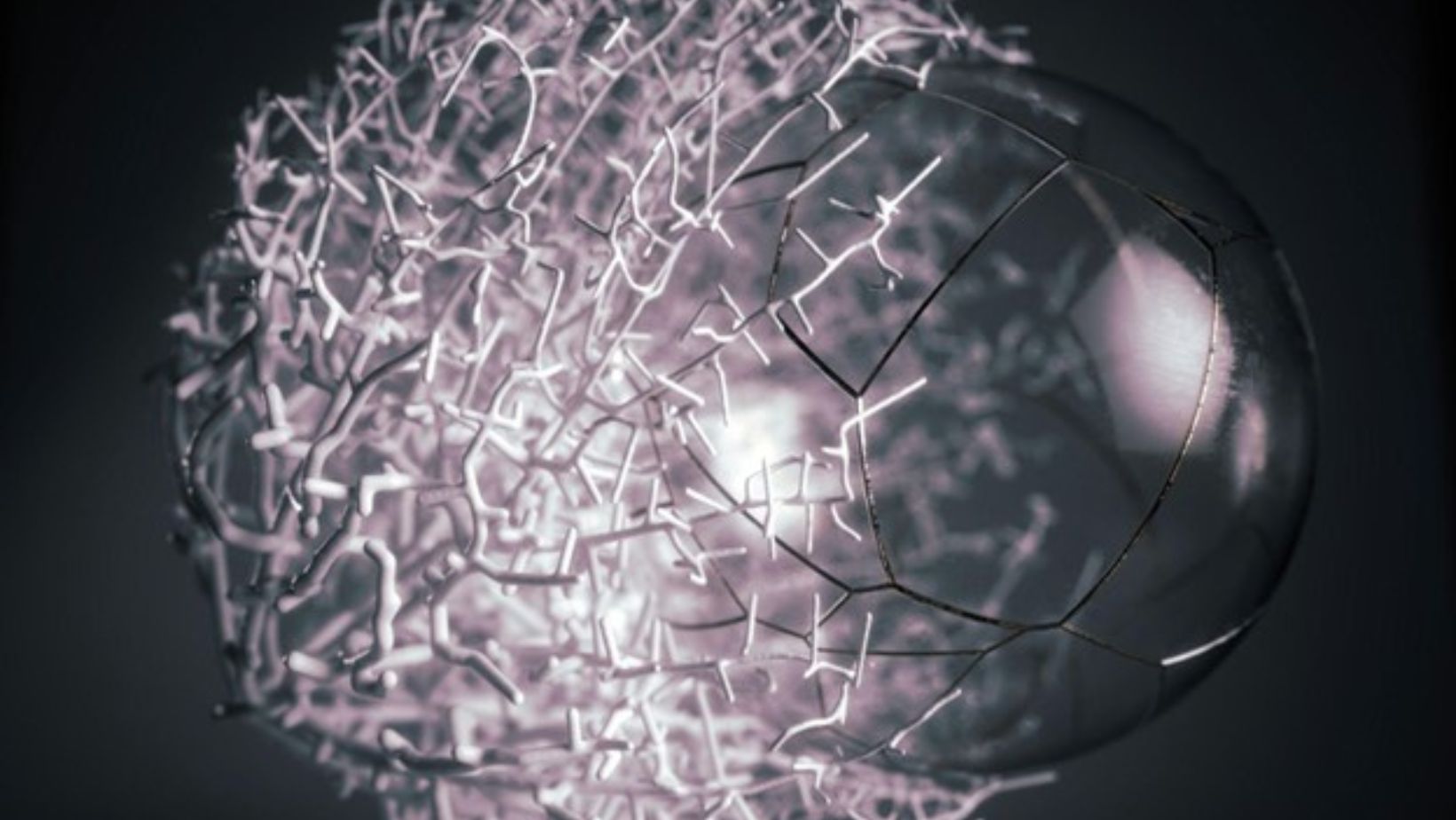
They can be maintained by such regulations as GDPR and HIPAA.
Technology Solutions for Secure Annotation
- Data Anonymization. Personal information is masked to prevent identification.
- End-to-End Encryption. Data transfers are secured to prevent unauthorized access.
- Access Controls. The access is provided only to authorized specialists working on the project.
Cloud-based platforms provide secure environments for annotation projects, helping companies comply with regulatory standards while protecting sensitive information.
Challenges Addressed by Technology
Here’s a summary of key challenges technology helps solve in data annotation:
- Slow Processes. Automation speeds up repetitive tasks.
- High Costs. AI tools reduce manual efforts, saving time and money.
- Inconsistent Quality. ML-powered error detection ensures consistent annotations.
- Workforce Limitations. Tools assist annotators in managing large workloads efficiently.
- Data Security. The technology ensures privacy and compliance with global standards.
Summing Up
With technology, the process of data annotation has become quicker and more efficient. While using AI-assisted tools and cloud solutions, you can streamline workflows and address various challenges.
As technology accelerates the process, human expertise remains essential for handling edge cases and ensuring context-based accuracy. By combining advanced tools with skilled annotators, companies can deliver high-quality datasets that power the next generation of AI models.